Improving Saliency Detection Via Multiple Kernel Boosting and Adaptive Fusion
Xiafei Zhou1 Zhi Liu1 Guangling Sun1 LinweiYe1 Xiangyang Wang1
Image and Video Processing LAB,Shanghai University1
Abstract
This letter proposes a novel framework to improve the saliency detection performance of an existing saliency model, which is used to generate the initial saliency map. First, a novel regional descriptor consisting of regional self-information, regional variance, and regional contrast on a number of features with local, global, and border context is proposed to describe the segmented regions at multiple scales. Then, regarding saliency computation as a regression problem, a multiple kernel boosting method based on support vector regression (MKB-SVR) is proposed to generate the complementary saliency map. Finally, an adaptive fusion method via learning a quality prediction model for saliency maps is proposed to effectively fuse the initial saliency map with the complementary saliency map and obtain the final saliency map with improvement on saliency detection performance. Experimental results on two public datasets with the state-of-the-art saliency models validate that the proposed method consistently improves the saliency detection performance of various saliency models.
Proposed Improving Model
Example of our method. (a) Input image; (b) initial saliency map generated using [11]; (c) complementary saliency map; (d) final saliency map.
Results
Visual comparison of saliency maps. In each example, the original image and the ground truth are shown in the first row, the saliency maps of DRFI-I, GMR-I, ST-I, HS-I, and RC-I are shown from the second to the sixth row in the odd columns, and the saliency maps of DRFI*, GMR*, ST*, HS*, and RC* are shown from the second to the sixth row in the even columns.
Quantitative Comparison
PR curves of initial, complementary, and final saliency maps on MSRA(left) and PASCAL-1500(right).
PR curves generated using Jiang’s and our regional descriptor and F-measures achieved by the weighted combination and our adaptive fusion method.
Citation
X. Zhou,Z. Liu, G. Sun, L. Ye and X. Wang, "Improving Saliency Detection Via Multiple Kernel Boosting and Adaptive Fusion," Signal Processing Letters, IEEE, vol. 23, no. 4, pp. 517-521, April 2016.
@ARTICLE{7163310,
author={ Xiaofei Zhou, Zhi Liu, Guangling Sun, Linwei Ye, and Xiangyang Wang},
journal={Signal Processing Letters, IEEE},
title={ Improving Saliency Detection Via Multiple Kernel Boosting and Adaptive Fusion },
year={2016},
month={April},
volume={23},
number={4},
pages={517-521},
doi={ 10.1109/LSP.2016.2536743 },
ISSN={ 1070-9908 },} .
Downloads
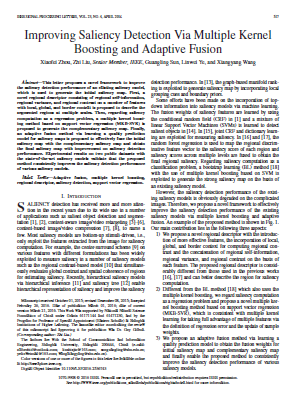 |
"Improving Saliency Detection Via Multiple Kernel Boosting and Adaptive Fusion" X. Zhou,Z. Liu, G. Sun, L. Ye and X. Wang, Signal Processing Letters, IEEE, vol. 23, no. 4, pp. 517-521, April 2016.
[Paper]
[MATLAB Code]
|