Superpixel-Based Spatiotemporal Saliency Detection
Junhao Lia Zhi Liua,* Xiang Zhangb Olivier Le Meurc Liquan Shena
School of Communication and Information Engineering, Shanghai University, Shanghai 200444, Chinaa
School of Electronic Engineering, University of Electronic Science and Technology of China, Chengdu 611731, Chinab
IRISA, University of Rennes 1, Campus Universitaire de Beaulieu, Rennes 35042, Francec
Abstract
In this paper, we propose a novel spatiotemporal saliency model based on superpixel-level trajectories for saliency detection in videos. The input video is first decomposed into a set of temporally consistent superpixels, on which superpixel-level trajectories are directly generated, and motion histograms at superpixel level as well as frame level are extracted. Based on motion vector fields of multiple successive frames, the inside-outside maps are estimated to roughly indicate whether pixels are inside or outside objects with motion different from background. Then two descriptors, i.e. accumulated motion histogram and trajectory velocity entropy, are exploited to characterize the short-term and long-term temporal features of superpixel-level trajectories. Based on trajectory descriptors and inside-outside maps, superpixel-level trajectory distinctiveness is evaluated and trajectory classification is performed to obtain trajectory-level temporal saliency. Superpixel-level and pixel-level temporal saliency maps are generated in turn by exploiting motion similarity with neighboring superpixels around each trajectory, and color-spatial similarity with neighboring superpixels around each pixel, respectively. Finally, a quality-guided fusion method is proposed to integrate the pixel-level temporal saliency map with the pixel-level spatial saliency map, which is generated based on global contrast and spatial sparsity of superpixels, to generate the pixel-level spatiotemporal saliency map with reasonable quality. Experimental results on two public video datasets demonstrate that the proposed model outperforms the state-of-the-art spatiotemporal saliency models on saliency detection performance.
The Model
Overview of temporal saliency measurement. (a) Input video frames; (b) example of superpixel-level trajectory; (c) frame-level and superpixel-level motion histograms; (d) frame-level and superpixel-level accumulated motion histograms; (e) inside-outside maps; (f) examples of salient trajectory (with red arrows) and non-salient trajectory (with purple arrows); (g) superpixel-level trajectory distinctiveness map; (h) trajectory-level temporal saliency map; (i) superpixel-level temporal saliency map; (j) pixel-level temporal saliency map
Results
Examples of spatiotemporal saliency detection on two videos from the VideoSeg dataset. (a) videos frames (shown with an interval of 20 and 10 frames, respectively, for the top and bottom example); (b) ground truths; spatiotemporal saliency maps generated using (c) SR, (d) CE, (e) QFT, (f) MB, (g) SP, (h) DCMR and (i) SLT, respectively
Examples of spatiotemporal saliency detection on two videos from the SegTrack dataset. (a) videos frames (shown with an interval of 4 and 10 frames, respectively, for the top and bottom example); (b) ground truths; spatiotemporal saliency maps generated using (c) SR, (d) CE, (e) QFT, (f) MB, (g) SP, (h) DCMR and (i) SLT, respectively.
Quantitative Comparison
Precision-recall curves of different spatiotemporal saliency models on (a) the VideoSeg dataset and (b) the SegTrack dataset, respectively.
Precision-recall curves of different maps obtained using our SLT model on (a) the VideoSeg dataset and (b) the SegTrack dataset, respectively
Citation
J. Li, Z. Liu, X. Zhang, O. Le Meur, and L. Shen, "Spatiotemporal saliency detection based on superpixel-level trajectory," Signal Processing: Image Communication, doi.: 10.1016/j.image.2015.04.014, Apr. 2015.
.
Downloads
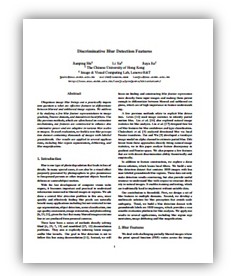 |
"Spatiotemporal saliency detection based on superpixel-level trajectory" J. Li, Z. Liu, X. Zhang, O. Le Meur, and L. Shen, Signal Processing: Image Communication, doi.: 10.1016/j.image.2015.04.014, Apr. 2015.
[Paper]
[MATLAB Code]
|